ChatGPT — why polish and packaging matters
Why we should focus on ChatGPT as a product and not just ML research and engineering side of things.
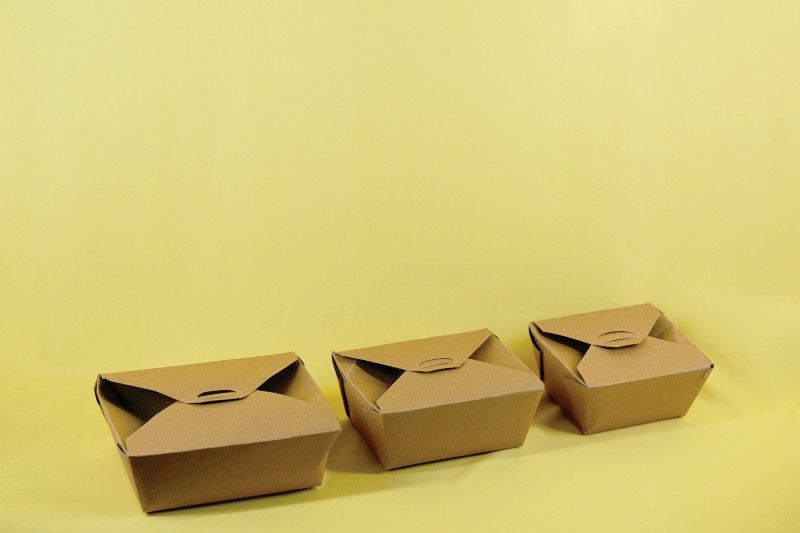
It has been some time since OpenAI released the ChatGPT GPT-3.5 powered service that rocked the world and generated an unseen before interest in Machine Learning among the less tech and ML savvy part of the populace.
And in the discussions about it we can see two main camps — one that says ChatGPT is a revolution in ML that brings us closer to AGI (Artificial General Intelligence) and the other that counters the notion saying that it is just repackaged stuff we’ve been using for quite some time and there’s nothing novel in it.
So who’s right between them? In my opinion both of those groups are at least partially correct but focus on the wrong things and miss the big lesson that the release of ChatGPT shows us.
And what that lesson might be? It is pretty simple but many times ignored, especially by the more tech and research focused — product design and polish matters greatly for the Average Joe. Both of those groups focus on the fundamental science and research side of things (progress towards AGI being the most obvious one) while ignoring ChatGPT for what it is really — an useful, accessible and well-made product with real-world use cases.
What ChatGPT is and is not
The basic thing many from the enthusiast camp don’t understand (considering they don’t fully understand the tech and science behind it) is that ChatGPT is not a fundamental revolution in ML theory and that it doesn’t really bring us in that regard closer to the holy grail of AGI.
As many from the ML/AI research community point out (here probably the heavyweight Yann LeCun being the most well known) there is nothing especially new or interesting to what makes up ChatGPT, both on the technical and the theory side. Fundamentally it is the well-known LLM (Large Language Model) architecture “spiced up” with an additional component which trained on human-labeled data helps the LLM choose answers which are more aligned with what a human would expect. An evolution at most, not revolution.
So are the experts like LeCun correct that ChatGPT will not bring us closer to AGI and doesn’t do much to help the progress in ML towards building better and smarter systems that could rival humans (or surpass them) in more and more applications? Yes and no.
The “no” from the above has been already explained but the “yes” is more interesting and where most people get it wrong. The enthusiasts say “yes” by misinterpreting the capabilities because of the lack of understanding of the underlying tech and theory. If something quacks like a duck it is a duck — if something speaks/writes like a human then it has human or at least close to human reasoning capabilities, which is of course incorrect (https://en.wikipedia.org/wiki/Chinese_room).
Why I agree with the “yes” is because of the buzz it creates around ML. And I make the assumption that the said surge of interest will translate into the following:
- More funds for research and development — both from private and public coffers
- A larger amount of people interested in the subject that will decide to start a scientific or engineering career in the field
- Companies and startups being created that like OpenAI will try to forge the theory into a usable product
We’ve seen the above happening with cryptocurrencies so there is some historical evidence that a similar thing should happen with ML. The only thing to watch out is for it not to get overtaken like crypto with scam artists and grifters that are just searching for a quick buck riding the hype train and eventually damaging the whole community around it along with the perception of the technology itself. Be wary of false prophets.
“It’s just packaging”
One of the most common arguments to downplay the significance of ChatGPT is that there’s nothing more behind it than nice packaging. Before I’ve explained that in many ways this is true as it does not bring nothing really new to the theory and understand of building reasoning-capable ML systems but the critical mistake is not appreciating the value of good packaging of a solution and a polished feature set when it comes to software, this can be seen especially from tech and research focused people.
At the end of the day the end user doesn’t really care about how a given application or piece of software works, as long as it brings him some value and is easy enough to use so that he doesn’t have to scour the Internet or a manual to operate it. An no, just because the 10 cryptic CLI commands you need to run in root-mode are easily searchable on SO doesn’t mean that the user-facing application is well made.
A great example of the above done right is the iPhone and in general the approach that Apple takes — creating easy to use, feature rich and well polished products so that people are willing to pay a premium for as they see that they bring value for them worth the difference in price compared to what the competitors offer. And money is one of the most fundamental metric of how much value the average person sees in something (absurd healthcare costs being a good example).
The iPhone just as ChatGPT wasn’t any kind of technical revolution — it used technology that was widely used and adopted before, but the way it was composed together was the real key of its success. Its birth essentially started the mobile era that we are a part of up until now and completely changed how we live our lives in many aspects (probably for the worse in some but that’s another discussion, the impact is what matters here). And so “just packaging” transformed some old boring tech used mainly by geeks into a consumer product with a global fanbase along with creating a new market for smartphones.
And what is important to understand is that the above success has started a lot of work and investment into many areas which brought in turn a great deal of progress to both software and hardware — such as the constant improvement in display technology, batteries and CPU performance to power ratio to name a few.
So my point of advice to all the engineers and researchers out there — do not ignore the importance of having a well designed and polished product towards the success of your area of interest. Even though it might not directly impact progress to what you’re interested in (e.g. designing Neural Networks to have cognitive capabilities closer to human) the ripple effect as history shows will be most probably have a net-positive in the long run.
What happens next?
We see now thanks to ChatGPT the thing mentioned before — there’s a big amount of interest generated in the underlying technology and more people are trying to come up with ideas how to package those into usable products that can be commercialized. It is a chance for the whole field to progress by obtaining more resources and investment which can be transformed into progress towards the long term goals like reaching AGI along with democratizing the access to amazing technology so that no one is left behind because of the gatekeeping of Big Tech. We need to show them support and avoid downplaying what they create as just shiny toys that bring nothing new to the table.
I encourage the creation of more products like ChatGPT — things that use the technology that’s out there and package it well to provide value for large swaths of people and remove the barriers of access that keep them from reaping the fruits of progress in ML. Machine Learning has a chance to become one of the most fundamental change factors in human history and the more restrictions for using it we remove the better.
Big kudos to the OpenAI team for getting us here (been a big fan of them since OpenAI Five which for me was jaw-dropping)! And I hope more and more people and companies will follow their path that will enable us utilize ML to make our lives better!
Thank you for reading!